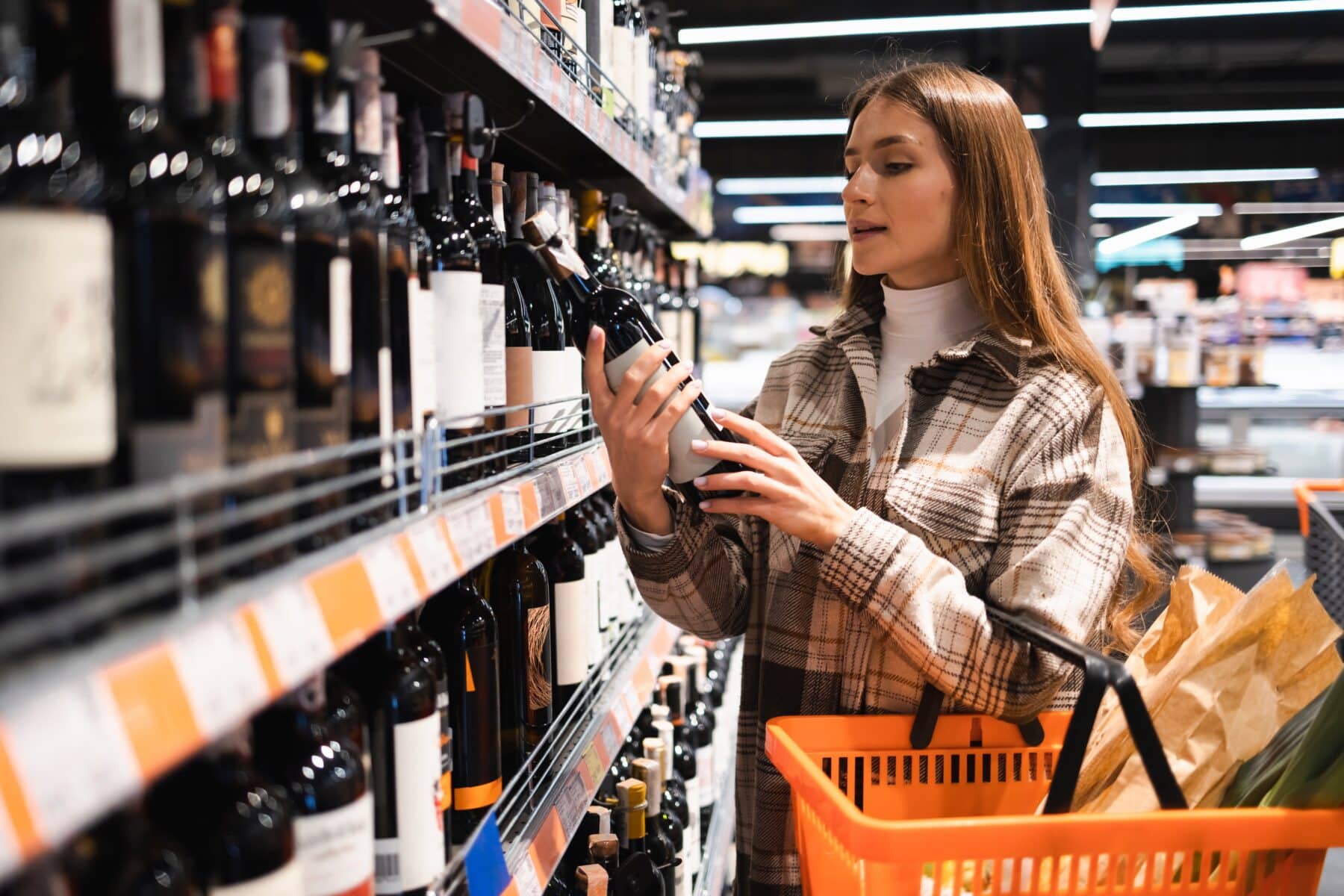
By Capri Brixey
Inside the four walls of the retail store, few categories are more complex than wine. For consumers, there’s an array of varietals from all over the world, each sold at wide-ranging — and to the consumer — seemingly random price points. For brands, there’s getting those bottles to the shelf, working through state-by-state regulations, distributor needs, and promotional limitations. For retailers, it’s knowing what to stock.
Using emerging technology, wine brands can simplify the process through AI-powered, data-driven optimization and decision-making. Producers can leverage predictive analytics to harmonize data from retailers, distributors, third-party sources, POS data and more. This includes, but is not limited to, macroeconomic and other influencing data that tee up strategic recommendations as granular as each store in their network.
As a result, wine brands can gain more control over where their wine goes and develop stronger relationships with their distributor and retailer partners, in addition to better managing supply and demand for future planning.
Understanding wine’s journey to the store
Currently, wine brands, big and small, need to jump through plenty of hoops to get products inside retailer doors throughout the country. To start, there’s a litany of governmental regulations to comply with, particularly regarding advertising and labeling wines, and how it’s imported and sold in certain states.
Brands must adjust their strategies on a state-by-state basis, factoring in unique rules before selling into retailers through a distributor network. Wine companies often work with several distributors, each with their own regional expertise and connections. However, distributors don’t just buy wine from a producer and sell it to the retailer. Distributors build a relationship with manufacturers to leverage sales materials, displays, and most of all, data and insights to help tell a story to the retailers.
Combined, regulations and distributor requirements can complicate how a wine company moves bottles of wine. Historically, brands have leveraged a matrix for each target state, distributor and retailer, and the data can get quite dizzying. Additionally, remaining inventory and pull-through rates have been used as performance indicators, even though those often do not reflect real preferences. Rather, they could reflect default performance through pushes to reduce latent inventory, or an incentive for display. This is where AI and predictive analytics can infuse levity and strategy.
Cutting through the complexity of the category
With so many entities at play and data sources from retailers, distributors, third-party providers and much more, brands can leverage AI to harmonize the data and deliver recommended strategies on what wines will sell best in what regions. In addition, AI can be modeled by brand teams to factor in state regulations and insights such as product availability within the distributor networks.
Machine learning models can look at data to see how one varietal is performing in a region in Ohio and how another varietal is performing in Texas. The AI can go even more granular to see how tastes change at individual stores or clusters in one region, too. This is tremendously important with store locations reflecting distinct differences in preferences depending on the location’s customer profiles. Retailers want to know distributors and wine brands will stock products that fit the tastes and demographics of the shopper attributes at each store.
Another advantage AI brings to the wine category is the ability to narrow in where choice may seem endless. Machine learning recommendations can help distill assortments down to the most essential for each store, presenting a measurable value to retail and distributor partners.
This pertains to pricing, too. AI can assist brands with recommendations on optimal price points for their wines by retailer. Value brands can fall under $10, for example, while premium brands can easily be $50 and up. AI can find and explain the price in that range that will deliver the best profit gains for a retailer and a brand.
Serving brands precise recommendations
To be sure, some industries fear LLM (large language model) AI outputs, questioning the accuracy and seeing a risk in its lack of explainability; however, AI-powered insights (different from LLM AI) should be looked at purely as an asset for wine brands.
With AI/ML, brand managers can input complex data and work with it to serve reliable recommendations to explore. Brand teams can plan endless scenarios for varietals, stores and regions and make strategic decisions in near real time that are not entirely reliant on historical data.
For wine brands, predictive analytics can enhance how the category delivers the right bottles to the right shelves, meeting regulatory compliance. Brands, retailers and distributors can work better together, using a better foundation as a starting point and further refine through execution.
Consumers will likewise respond to assortments aligned to their preferences, as retailers create efficiencies and consumer delight within their existing spaces. Simply put, machine learning and predictive analytics uncorks opportunities for wine brands that they’ve never seen before.
__
Capri Brixey is EVP of strategy consulting at Insite AI, bringing extensive strategic leadership experience from both retail and supplier roles in the consumer goods industry. Most recently with The Coca-Cola Company, Capri has led small and large-store teams, across multiple routes to market, channels, categories, and segments in the industry. Recognized in 2022 as a Senior Level Leader for Top Women in Convenience, Capri has also been recognized for her leadership in collaborative/joint business planning with top retailers across multiple channels/formats.
ck unprecedented opportunities.