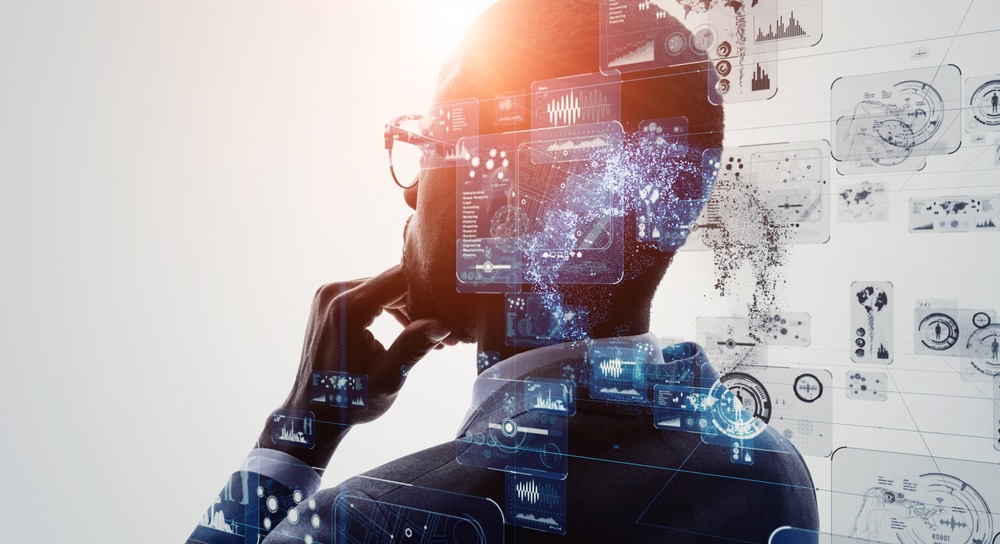
Written by Gopalakrishna Tadiparthi, SVP of machine learning and artificial intelligence
Amid all the excitement and buzz circling around AI, it might be a bit sobering to learn that most AI projects fall short of their goals.
Gartner originally laid claim that 85% of AI projects fail, largely due to erroneous data. Another more recent Gartner study predicts that half of AI deployments in the finance sector will be delayed or shut down by 2024.
In retail, the success rate of AI projects can be similarly soft and there are a few reasons why — notably data and a lack of education around how to use AI. Surely, erroneous or unclean data can hinder projects, but also brand teams that aren’t empowered to learn and grow with the technology can stall a project’s success. Some companies come to the technology with a “set it and forget it” attitude, not embracing the pivotal partnership humans play in making AI-powered insights and predictive analytics thrive.
Truthfully, there is no single defining reason why AI projects fail, but as consumer goods companies implement AI, there are some common missteps and reasons. Here are three to watch:
1. Limited or under-used data
Companies receive and purchase data from many vendors and retailers. The power of AI and ML is in the fuel (i.e., the quality of data along with the quantity of data). Since the data is from disparate sources, they need to be harmonized. The additional efforts in harmonization and the unknown value of the new data are preventing companies from using the data.
In machine learning and AI, there’s never enough data. A primary reason AI projects fail is when companies don’t feed the machines enough quality sources of information to make accurate decisions and recommendations. Companies need to keep shoveling the furnace coal and fueling AI algorithms so that they continue to learn and adjust in real time.
Keeping the data clean is also important. Many AI programs struggle when an AI solution provider removes a client’s data from its internal IT infrastructure. Insite AI works directly within a client’s cloud.
Machine learning platforms need to fit within a brand’s architecture, using their internal data sources inside their framework. Keeping the technology in-house ensures clean and effective results.
2. Failure to customize AI models
Many companies focus on obtaining the latest and greatest technology. Technology FOMO is a real thing. However, teams must focus on the business problem they are solving for — then pick the appropriate model to solve the problem. Often, if a business issue is unique to a brand, the company will need a customized AI model. And, to get the most out of a machine learning tool, CPG teams throughout the organization need to learn the customized model inside and out to address their specific business needs.
For example, marketing teams can identify brand-switching behaviors that inform campaigns to reduce leakage. At the same time, finance teams can forecast demand around products to influence budgets. There are many examples, but the key is for each user to identify the variables that will help grow their forecasting needs.
For instance, if a CPG is standing on the principle of always delivering competitive pricing. Teams need to learn how to use the models and focus on the variables to help forecast a competitive pricing strategy. Then, CPGs need to think about how to derive insights from the models to get the most out of the learnings.
3. Lack of continued learning and resources
Brands cannot be complacent because they implemented an AI system. They have to scale the process and embrace the data-driven decision-making culture. Just as there’s never enough data for an AI program, brands can’t let up on feeding the machines insights. Of course, this requires a steady diet of internal resources and investment.
Brands need to put a plan in place that manages how teams use AI long term. Companies that go heavy early with the modeling can weaken results later if they’re not continuing to feed the AI on an ongoing basis. Brands need to understand and plan the time and costs associated with a more efficient and effective use of AI.
As the AI algorithms continue to learn and deliver insightful, accurate predictive analytics, CPGs will grow their brands and develop stronger categories overall. Brands that plan accordingly can use AI proactively, getting out in front of large events that can alter a category, rather than using the tools in react mode.
The success of an AI program inside a brand’s business requires data analysts and category teams to be empowered to learn the models and leverage the insights. C-level executives should build a culture around AI that ensures the technology keeps learning and so do the brand teams working directly with it.
Learn more with Insite AI
Insite AI helps tackle common pitfalls that companies face when working with AI models. With a focus on knowing consumer brands at their core, Insite AI harmonizes key data and uses it to enrich the training process of the AI models being used. Insite AI partners with brands to create custom solutions that solve their unique business problems and is transparent in how it communicates the model training process and explanation of the AI/ML methodology.