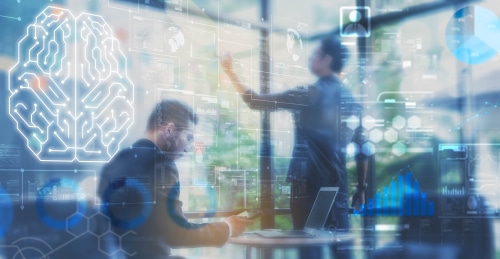
Consumer preference and behavior changes lead CPGs to renovate and rationalize their portfolios while satisfying retailer needs. But being mindful of how demand transfer affects both sides allows for optimal assortment mixes and accurate transferable demand calculations.
Making Sense of Transfer Demand to Unlock CPG Agility
Consumer needs are changing fast and a new innovation is equally as likely to come from a well-funded technology startup as it is another CPG. Consumers are hungry for newness and have growing appetites for plant- or animal-free products, purpose-led brands, and sustainability. These changes have led CPGs to renovate and rationalize their portfolios, while retailers are seeing a growing number of SKUs from which to choose and are increasingly shifting to smaller store formats, meaning even less room on shelves. How do you rationalize ranges at scale without losing sales to competitors? One of the critical factors to follow closely is transferable demand.
Put simply, transferable demand is about understanding the effects of listing or delisting products. It considers how demand moves between products, brands, and even categories.
How can your CPG create optimal assortment mixes and calculate transferable demand accurately?
And how can you do it across millions of different brand and SKU combinations? If you’re able to get it right, you’ll work with the confidence to make optimal range and pricing choices in a matter of hours or days instead of weeks and months. Major assortment, pricing, and range delisting decisions are going to be far reaching across the enterprise, and their monetary effects could go one of two ways. Historically, the performance of a go-to-market strategy that lists a new or removes an existing SKU or product won’t be known until weeks or even months ahead. But, the right intelligence on transfer demand can ensure ranges are evolved safely, without losing category turnover or share.
Previously, optimizing an assortment and predicting transferable demand was a computational impossibility. Picture this scenario: you need to choose 300 products out of a possible set of 500. There are more ways to choose 300 products out of 500 than there are atoms in the entire universe.
So what do you do and how do you predict transferable demand?
An AI solution that can sit within your organization’s private cloud, looking at data from multiple sources, is the start. including sales and category data down to the most granular EPOS information. Inputs can also include everything from sales and category data, EPOS metrics, 3rd-party data, shopper behavioral insights, and 3D spatial information and planograms.
Across tens of thousands or millions of households, the optimal solution looks at the level of item loyalty within a particular category; for example, what percentage of shoppers are buying the same product every week. It identifies products that shoppers are willing tosubstitute and where shoppers switch brands regularly or buy from an extended repertoire.
It also looks at a product’s importance to customers in terms of total value, far beyond simple metrics such as rate of sale per store. For example, a niche, slow-selling SKU could be so important to some shoppers that its delisting could lead them to move their entire spend to other brands or even retailers. But how can you predict these results ahead of decisions being implemented?
Using an output that makes decisions at scale with accurate forecasts on the share of sales that would be reallocated to other brands in the brand portfolio and the share at risk of being lost, either to other brands or from the category entirely, is the holy grail of the CPG’s toolset.
The importance of consumer decision trees (CDTs)
Consumer decision trees are a visual representation of how consumers choose products according to different need states. Products are grouped into clusters that share similar product attributes, and it’s within these clusters that product switching is most likely to take place. The different product attributes are shown in a hierarchy: by brand, price point, taste, texture, ingredient lists, pack sizes, and more. For example, in the growing dairy-free milks category, there are variables such as soy, almond, oat, chilled, ambient, sweetened, non-sweetened, organic variants, barista variants, and flavors.
Using consumer decision trees, an AI solution can help CPGs identify demand transfer between different clusters of consumer need states, identifying how the introduction of a new product or range will interact with existing products. Opportunity gaps within a category can be quickly identified and the transfer demand from other products accurately predicted. For example, if you’re a CPG in the highly-competitive laundry category and you launch a sustainable, earth-friendly brand, a good AI tool will be able to predict the level of possible brand cannibalization, as well as the opportunity size in gaining share from competitors.
Unlike many off-the-shelf AI platforms, Insite AI is highly adaptable to meet a CPG’s specific business challenges. We understand that your enterprise needs to be agile in meeting consumer needs and reacting to a volatile economic climate while maintaining growth and dealing with the complexity and limits of the supply chain. With advanced AI analytics, you will have the organizational capability and intelligence needed to move faster and smarter.