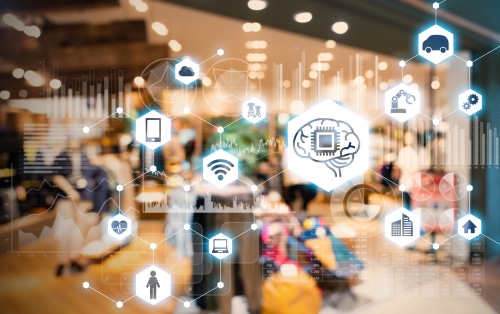
In this episode, we’re talking about how AI is being used with both CPG companies and retailers to better predict consumer behavior.
Listen to our latest podcast episode on predicting shopper behavior. In this episode, we discuss key strategies for optimizing at-shelf availability, distribution, assortment, and total portfolio optimization.
First appeared here: https://www.foodprocessing.com/podcasts/food-for-thought/buyer-behavior-artificial-intellgience/
Transcript
Erin: Welcome you to the Food For Thought podcast.
Ryan: Thank you very much. I am very pleased to be here.
Erin: Let’s start talking a bit about what Insite AI is all about. What does the company do and who is your end-user?
Ryan: Yeah, thank you very much for the forum. Insite AI, we deliver artificial intelligence solutions focusing on hyper-localization, so solutions that are really tailored or customized to specific problems or platforms that customers are looking for or problems they’re looking to solve. We primarily focus on CPGs. Every now and then, we do connect with retailers, but our primary customer is the CPG, and we do a wide range of things in the artificial intelligence space for them.
Erin: With all of the mountains of data out there, it can definitely seem overwhelming with what to do with it and what direction to take with it. Can you talk about that a little bit more and how InsiteAI incorporates the data that CPG companies are feeding into it?
Ryan: This is actually a question that is one of the first hurdles that we have to overcome when we’re dealing with CPGs. Usually, there is a fairly limited data set. We know that there’s a universe of data out there, but what’s actually usable and complete and clean is a fairly limited data set. So one of the things that we’re finding is that as we start to bring in these mountains of data, one of the first things that we really have to align on is what is clean and complete and what is very accessible. The second part really then factors into the remainder of the mountain, which is all of these really small data points.
A really good example that’s coming out very frequently right now is things like mobility, right? Like external data that can really enrich some of the answers from a predictive system that allows you to kind of drive and do different calculations than you would be able to do if you didn’t have it. But you have to figure out how to incorporate that, how to plug it in where it makes most sense, what type of calculations can leverage it best. And when we’re talking specifically in the food production industry or food services industry, that type of data is hugely important to understand where are people gonna be, what are their patterns, things like that.
With COVID as well we’re seeing a lot of that data that can also be incorporated in understanding, “Will people leave their houses? Will people go to specific restaurants? What type of behaviors will they exhibit?” And some of those COVID data sets will drive, you know, kind of these knock-on forecasts or knock-on patterns that will essentially affect that consumer behavior.
There is a mountain of data out there that the best approach if you’re a CPG or food manufacturer is to analyze each of those data sets against that core base data set that you have. Really start to understand what the value of those components are to your specific business. Artificial intelligence and leveraging data is a little bit like alchemy in the sense that when you’re developing a specific potion, it’s so custom that as you start to try and take that somewhere else, sometimes it just doesn’t work. A data set that was very usable and sensitive to a specific partner is not as usable or sensitive to another partner and essentially it can take you backwards in accuracy. The data really needs to be deciphered and tested. And from that you need to drill down into those couple needles within the haystack of external data within that mountain that can really support the answers in a positive way and support your accuracy increase.
Erin: I like how you mentioned the alchemy aspect. You used a term that I’m not as familiar with that I’d like you to elaborate on. What exactly is clean and complete data?
Ryan: Thank you for giving me the opportunity to elaborate on that because this is something that every single company, partner, student, anyone that’s leveraging any artificial intelligence to drive predictions or understand trends or do analytics is going to come up against. And essentially, the cleanness of data really comes down to the fact of, “Is everything in the right column? Is everything categorized correctly, spelled correctly, do all the characters align the same?” Because what you’re using in artificial intelligence is really being able to crunch a bunch of numbers in a very small amount of timeframe.
You’re crunching a mountain of data, numbers, and attributes and you’re correlating and calculating everything. To get the best answers, everything in there needs to be in an ideal state – as clean as possible. And that way, you don’t get anomalies or mathematic or statistical outliers driven by formulas. Because if you’re getting those, you want them to be relevant and based on the math you’re using. You don’t want them to be irrelevant based on something that’s unclean, that sends accuracy down or confuses the system or the output.
The second piece of that, which is the completeness, is something that we deal with a lot, especially in CPG. Sometimes we get data sets where we might only have a certain number of brands, or we might only have a certain amount of markets, or we might only have data for a specific period. Where you have a projection or a prediction that needs to be executed, but you only have a slice or a sliver without having the complete picture. And in some of these cases, you can kind of work around it.
We’re dealing with a specific set of brands and we know our brand really well. We have all of our data as well as some retailer data and some of the market data, then we can start to put certain pictures together. But, for example, if you’re dealing with very localized, specific, fragmented data such as receipt data, partial loyalty data, or partial sales data, that’s where it becomes a little more tricky because you can start to get these signals that actually send you in the wrong direction.
When we talk about the alchemy piece of it, that’s really one of those things where you need to understand not only the mass behind it and how to actually approach the artificial intelligence. You really then need to understand what your raw materials are to play with so you can produce the best results out of those raw materials versus just applying a methodology in a cookie-cutter fashion.
Erin: I know in doing the research for this particular episode that Insite AI’s tool can help the food processors listening to this episode with behavioral predictions. Walk me through what that’s all about.
Ryan: The principles around artificial intelligence can be achieved through lots of different means. You start to crunch the data, and you’re not just dealing with quantitative data, you’re dealing with qualitative component, but you realize you not only understand kind of what’s going to happen, but you can start to understand the fragments and the segments and the clusters behind what’s driving that future or that prediction.
And I think for us, that’s what really allows us then to start to understand behavior in a very segmented fashion. We are seeing so many microsegments that have popped up. And it’s driven by a combination of different trend timelines specifically driven by the current external environment. We’re seeing timeline-driven components. We’re seeing buying behavior or shopper behavior components as well. You’ve got an ecosystem that, prior to this, was fairly understood. Now what we’re seeing is that ecosystem that we understood is not only changing in these short bursts, but they’re also fragmenting within those changes.
Just to understand that behavioral component of it is really important. That behavioral component of is really telling you which groups of shoppers or consumers are changing, how quickly they’re changing, what groups they’re falling into. And then ultimately, how can you then focus on that level of understanding to then predict their buying pattern? And this, we believe, is going to become hugely more important for really everyone in manufacturing and retail to understand because it means that some of these behaviors are going to be short-term and some of them are gonna be long-term. And you really have an ability to actually influence and drive and set some of those behaviors within these, kind of, short burst trend cycles. And so that’s one of the huge benefits that we’ve seen. And it really is, you know, all about understanding your customer or your consumer at the level in how they’re thinking about the category they’re purchasing in.
Erin: I love what you’re talking about with helping identify and isolate potential short-term or longer-term behavior. If we’ve learned anything in the last year, it’s how disruptions can so severely impact things across the entire supply chain. Can this tool able to help with forecasting when disruptions occur?
For instance, let’s say you are a food manufacturer and you’re looking at your consumer data, you can see that, “We make this particular brand of oat cookie, or we use a certain kind of oat in our product formulation.” Is this the kind of tool that processors can use to say, “We can see people in the Midwest or people in the East are really buying a lot of this particular oat cookie, we need to make sure that we have ordered even more of the oats that we need?” Is that how this tool can help processors?
Ryan: Yes, it’s absolutely one of the ways that kind of leveraging these techniques and these tools you can benefit. First, understanding, “Hey, if I have issues further up the supply chain or sourcing components, I can start to take a look at those and analyze, forecast, and game plan for when I’m going to have that I know.” That leads in to having a trickle-down strategy to say, “Okay, now, I have my kind of core materials and understand what I’m gonna have. Here’s what I can do with those.” Absolutely, we can predict based on those materials, what we know, what we’re going to be able to do with them, and ultimately, what results we’re going to get through the demand and the purchasing.
One of the most interesting things that I’ve seen and been working on over the last couple months is not only just understanding the disruption from the sourcing component or disruption from the global pandemic component where things are happening and we’re trying to figure out, “Okay, what do we have? What can we do?” It’s also from the standpoint of saying, “Hey, you know, if there is a major area of our business that we didn’t see coming, that we didn’t necessarily have that strategic plan for, leveraging the artificial intelligence and the speed and the scenario planning that you can do with tools like this really gives you the ability to essentially disaster control or crisis control your environment.”
If you have a system in the background and you have potential situations where you can’t source materials for certain products, or you can’t now deliver certain products to the store, what this will allow you to do is understand the issue at hand. It also lets you take a look at a larger kind of portfolio level and say, “What other things do I have and how should I approach leveraging those other, products, brands, avenues, and where? Where should I leverage them? If I have an issue where there’s a certain batch of product that I can’t use or I can’t deliver, do I just shift things from other places?” And what’s the impact to that, right? That’s kind of one view or thought process.
There’s also the situation of, “I just can’t get anything. I’ve got a ship that’s stuck in Panama. I’ve got a whole product line that’s going to go down or potentially a whole brand that’s gonna go down for a period of time. What should I put in? What would be the best bet from a retailer perspective? What would be the best bet from a CPG profitability or volume perspective?” Being able to look at that further in real-time and to then say, “Well, is that the strategy I wanna execute? Do I want to push the customers or the consumers one way or another because I now know what the outcome of this crisis is if I just do the normal thing? Maybe I can start to look at multiple different types of outcomes, where I’m doing something non-traditional. I’m pushing them into a different area.”
The last part would be competitive information. You can also gain some competitive intelligence. The timeframe you’d will probably a lot shorter because competitors aren’t going to tell you what’s going on, but at the retail level, you’ll start to understand some of the challenges going on. When you have systems that can move very quickly and analyze in real-time or take in non-traditional data or qualitative data, you can start to then do the same thing with competitive landscape data. This helps you to understand, “Okay, my competitor’s going to have a supply issue. My competitor’s going to have a sourcing issue. How do I capitalize on that? How do I go to a retailer and partner with them or make sure I’m satisfying the consumer with other products that potentially I wouldn’t have had that opportunity if there wasn’t an issue with that competitive landscape?”
There’s so much that can be wrapped into that with what you can do. And it really is all about understanding what’s happening in a moment and being able to not only project, you know, the outcome, but also to strategize along the lines of, “Hey, listen, you know, something’s going wrong. Let’s at least see what the best outcome we have in line with the strategy that we actually want to execute.”
Erin: Are there any trends or behaviors that your teams or clients are picking up on that you can share? I don’t know if that’s competitive information, but is there anything that you guys have noticed that you’re able to share?
Ryan: I think the biggest thing that we’ve seen that’s been happening in the market is the formulation of some of these microsegment behaviors that are actually changing the way that people buy products in stores, but more importantly, really how they buy products in channels. What we are seeing a lot of is really the last year or so has trained the new generation that they don’t need to do things the way that they were doing before. And so some of the anticipated behavior is that things are gonna go back to normal to a certain extent. And in some cases, that is going to be true.
I think one of the biggest challenges and biggest insights that we’ve been able to reveal is that you really have to understand the segment of the shopper in your specific category and how it’s changed over the last 18 months to be able to actually secure that shopper with long-term loyalty. If you think classic brands back in the day, big brands that everybody remembers, where you’re either one thing or another, everyone kind of picks a side and it’s very polarized and it becomes very loyal to products, it’s no longer really that way. The landscape’s much more diverse, and there are a lot more options and a lot more areas where someone can purchase. And so what we are really seeing is that those behaviors are being solidified right now. And essentially, consumers have had the opportunity to test to be part of different behavioral groups, to test different shopping experiences.
This is all opportunities for the retailers and the CPGs to actually secure new customers that they wouldn’t have had access to before. Because of that, now we’re in a situation where the biggest or largest advantage that a CPG can get for itself and a retailer as well is to really deep dive and understand those segments and really understand what’s making those segments tick, how they’re buying, what the makeup is so that they can then formulate those larger segments, push people back into the kind of macro clusters where they were before, where you can manage them more efficiently. Otherwise, these fragments are going to continue and it’s going to become very, very difficult to satisfy large groups of customers because they all are going to buy in these really fragmented and separate ways.
We are seeing a lot of that across multiple categories and partners that we work with. And I think it’s still changing and there will be some settling that happens over the next two years or so. But it is going to look, we believe, fundamentally different. And the current consumer understanding and different roles and constructions that we have previously adhered to we believe are gonna be broken and changed. And whoever figures that out first, you know, and embraces it and strategizes against it is going to see significant wins.
Erin: You have definitely given our listeners a lot to think about and ponder on and so much information about what Insite AI’s tool can help them with. So last question for you is, for our listeners, if anyone wanted to reach out, learn more, maybe hop on board and use this tool as well, how could they do so?
Ryan: Yeah, the best way is you can contact me directly. My email is ryan@insite.ai. I’ll be happy to connect with you, connect you with the right team members. You can also go onto our website and get an understanding of what we do. But what we find is the best way to understand about this is to talk to us. Genuinely bring the challenges that you have. We are going to listen. We are going to break that down, really understand where we can help, really understand where it’s better for you to do things yourself or with other vendors, and approach it in just a really pragmatic way to make sure that you get the best results. So engage with us with your questions, and we’ll genuinely help you try and resolve those challenges, whether it’s something small or something large.